Angola Joins Growing List of African Countries to Remove Fuel Subsidies
Angola eliminates diesel subsidies, following IMF advice, to free up $3 billion for health and education budgets, sparking concerns over transport costs and economic impact.
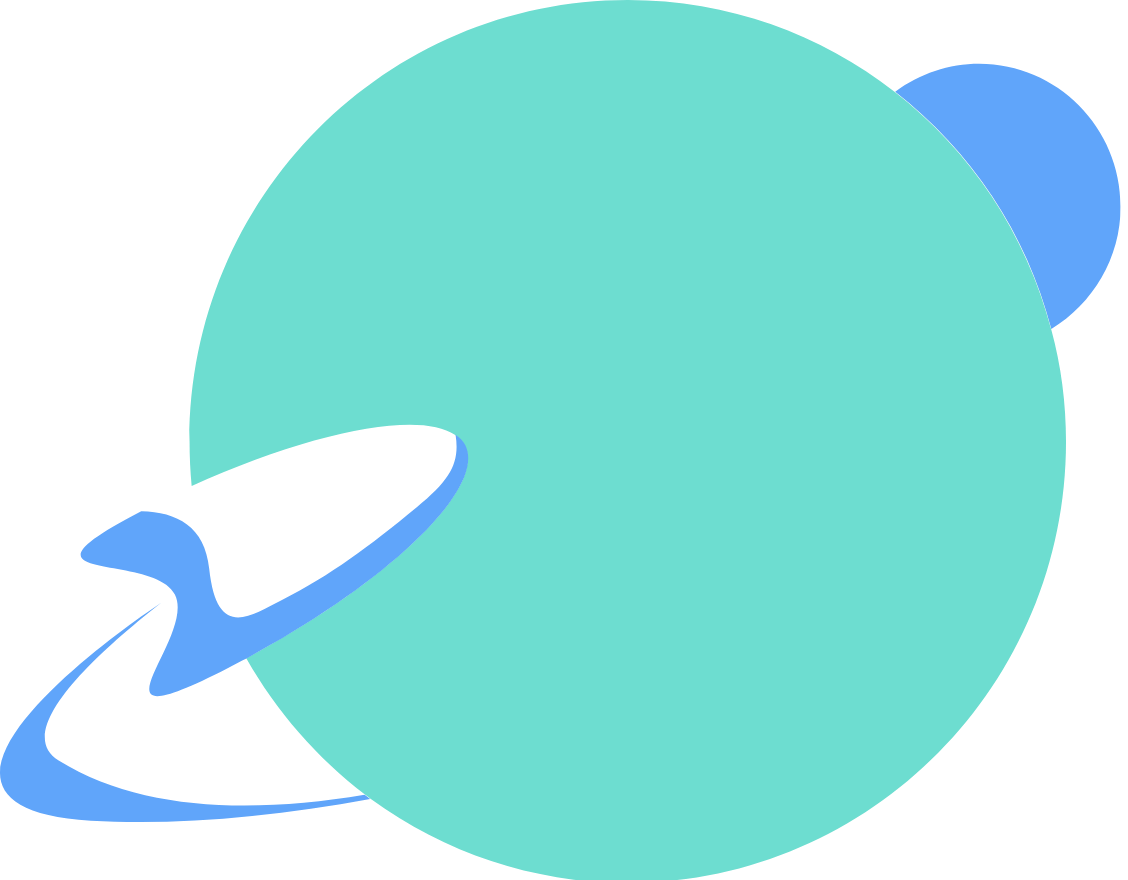
Riley King
A recent paper by Google and UC Berkeley researchers has sparked debate in the AI community, with some commentators hailing the proposed "inference-time search" as a new AI scaling law. However, experts are skeptical about its practical applications, citing limitations and high computational costs.
AI scaling laws describe how the performance of AI models improves as the size of the datasets and computing resources used to train them increases. Until recently, pre-training was the dominant approach, but two additional scaling laws, post-training scaling and test-time scaling, have emerged to complement it. The new paper proposes inference-time search as a potential fourth law, which involves generating multiple possible answers to a query in parallel and selecting the best one.
The researchers claim that inference-time search can boost the performance of a year-old model, like Google's Gemini 1.5 Pro, to surpass OpenAI's o1-preview "reasoning" model on science and math benchmarks. According to Eric Zhao, a Google doctorate fellow and co-author of the paper, "by just randomly sampling 200 responses and self-verifying, Gemini 1.5 beats o1-preview and approaches o1." However, experts argue that the approach works best when there's a good "evaluation function" – in other words, when the best answer to a question can be easily ascertained.
Matthew Guzdial, an AI researcher and assistant professor at the University of Alberta, notes that most queries aren't that cut-and-dry, making inference-time search less useful in many scenarios. "If we can't write code to define what we want, we can't use [inference-time] search," he said. Mike Cook, a research fellow at King's College London specializing in AI, agrees with Guzdial's assessment, adding that it highlights the gap between "reasoning" in the AI sense of the word and our own thinking processes.
Cook argues that inference-time search doesn't "elevate the reasoning process" of the model, but rather works around the limitations of a technology prone to making confidently supported mistakes. "Intuitively, if your model makes a mistake 5% of the time, then checking 200 attempts at the same problem should make those mistakes easier to spot," he said.
The limitations of inference-time search are likely to be unwelcome news to an AI industry looking to scale up model "reasoning" compute-efficiently. As the co-authors of the paper note, reasoning models today can rack up thousands of dollars of computing on a single math problem. The search for new scaling techniques will continue, as the industry strives to develop more efficient and effective AI models.
In conclusion, while the proposal of inference-time search as a new AI scaling law is an interesting development, its practical applications and limitations need to be carefully considered. As the AI community continues to explore new scaling techniques, it's essential to prioritize approaches that can be effectively integrated into real-world scenarios, rather than relying on computationally expensive workarounds.
Angola eliminates diesel subsidies, following IMF advice, to free up $3 billion for health and education budgets, sparking concerns over transport costs and economic impact.
Microsoft makes significant AI investments, creating a new engineering group, relaunching Copilot Chat, and bundling Office AI features into Microsoft 365, hinting at a future of automated services and revenue opportunities.
A new amendment bill in Kenya seeks to increase core capital requirements for banks, potentially forcing 24 banks to close, while Amazon develops a new AI-powered Alexa and Nigerian finance employees express salary dissatisfaction.
Copyright © 2024 Starfolk. All rights reserved.